GlassMindNet
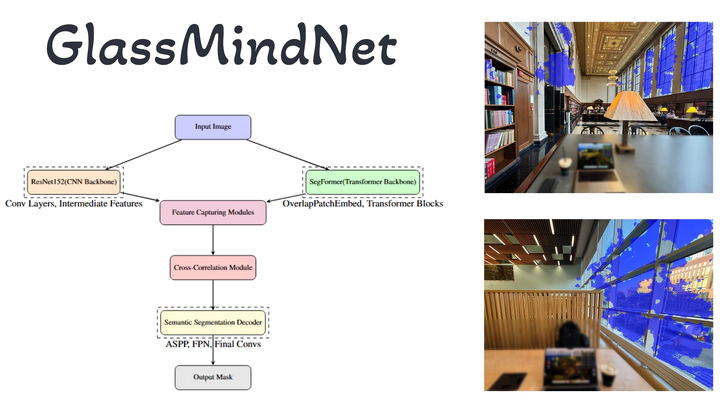
The increasing prevalence of glass curtain walls in modern architectural designs poses significant challenges for vision-based autonomous systems, including those used in vehicles and drones. These challenges arise due to the transparency and lack of clear boundaries inherent in glass surfaces, which often confound traditional image recognition methods. In this work, we propose GlassMindNet, a novel deep-learning-based framework for precise and robust detection of glass surfaces in diverse environments. Our approach leverages semantic relationships between glass surfaces and their surroundings through a custom architecture combining feature capturing, cross-correlation mechanisms, and a semantic segmentation decoder. GlassMindNet integrates state-of-the-art transformer backbones (SegFormer) and ResNet152, enhanced by novel attention modules, including Feature Capturing and Cross-Correlation modules, to effectively merge spatial and semantic features. The model is trained and evaluated on a curated dataset of urban and architectural scenes, achieving significant improvements in Intersection over Union (IoU), F-beta score, and Mean Absolute Error (MAE) compared to existing methods. Through extensive experimentation and ablation studies, we demonstrate the effectiveness of our approach in handling edge cases, such as reflections, occlusions, and varying lighting conditions. The proposed model achieves an IoU of 0.89 and an F-beta score of 0.95, indicating its capability to detect and segment glass surfaces accurately. This work advances autonomous navigation and safety systems by addressing a critical gap in the semantic understanding of challenging visual environments.